NAS-X is a new method for fitting discrete and continuous latent variable models that combines smoothing sequential Monte Carlo (SMC) with reweighted wake-sleep (RWS). NAS-X's gradient estimator is much lower-bias and -variance than competing methods, resulting in state-of-the-art sample efficency and learning.
RWS methods fit parameters by descending Monte Carlo estimates of gradients of the marginal likelihood. For sequential models, SMC is often the Monte Carlo sampler of choice. Unfortunately, standard filtering SMC only incorporates information from past observations, leading to biased gradients and poor-quality proposals and models. NAS-X instead uses techniques from SIXO to approximate smoothing SMC, which, in theory, allows the true posterior to be recovered.
In our paper, we evaluate NAS-X in an array of challenging tasks including discrete latent variable modeling and fitting large mechanistic models of neural dynamics. We found that NAS-X substantially outperformed both SIXO (smoothing but no RWS) and NASMC (RWS but no smoothing) on all tasks.
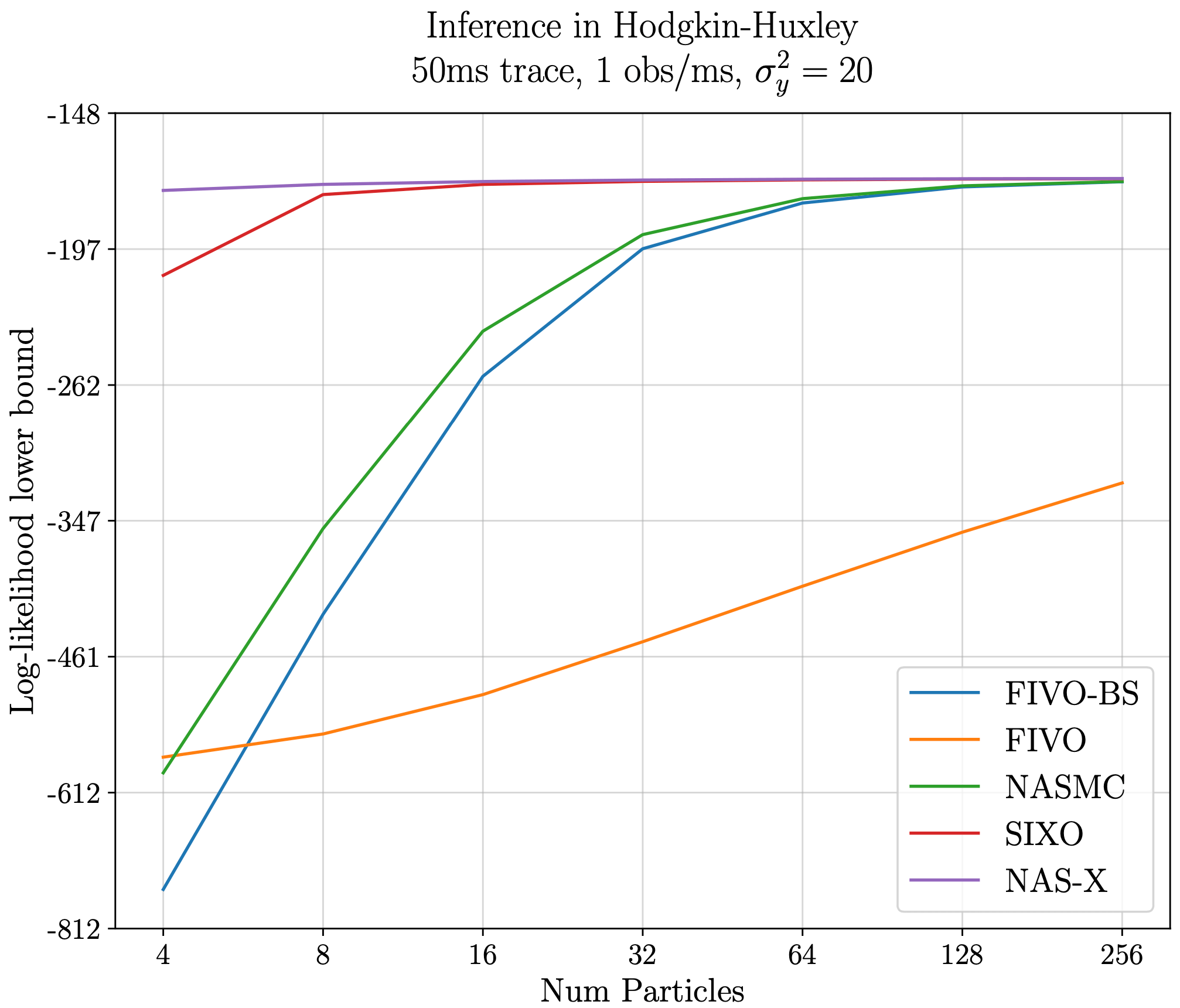
For example, this plot shows that on the mechanistic modeling task NAS-X is 4 times more particle-efficient than SIXO and at least 64 times more particle-efficient than NASMC. We believe these gains are the result of NAS-X's gradients having substantially lower bias and variance than competing methods.

Make sure to come visit our poster at NeurIPS, #1219 on Tuesday, December 12 at 5:15 PM!